Improve data quality
Boost trust in data and process
Increase reliability and data quality throughout your entire data lifecycle, so users have confidence in their data and the business has confidence in process and analysis.
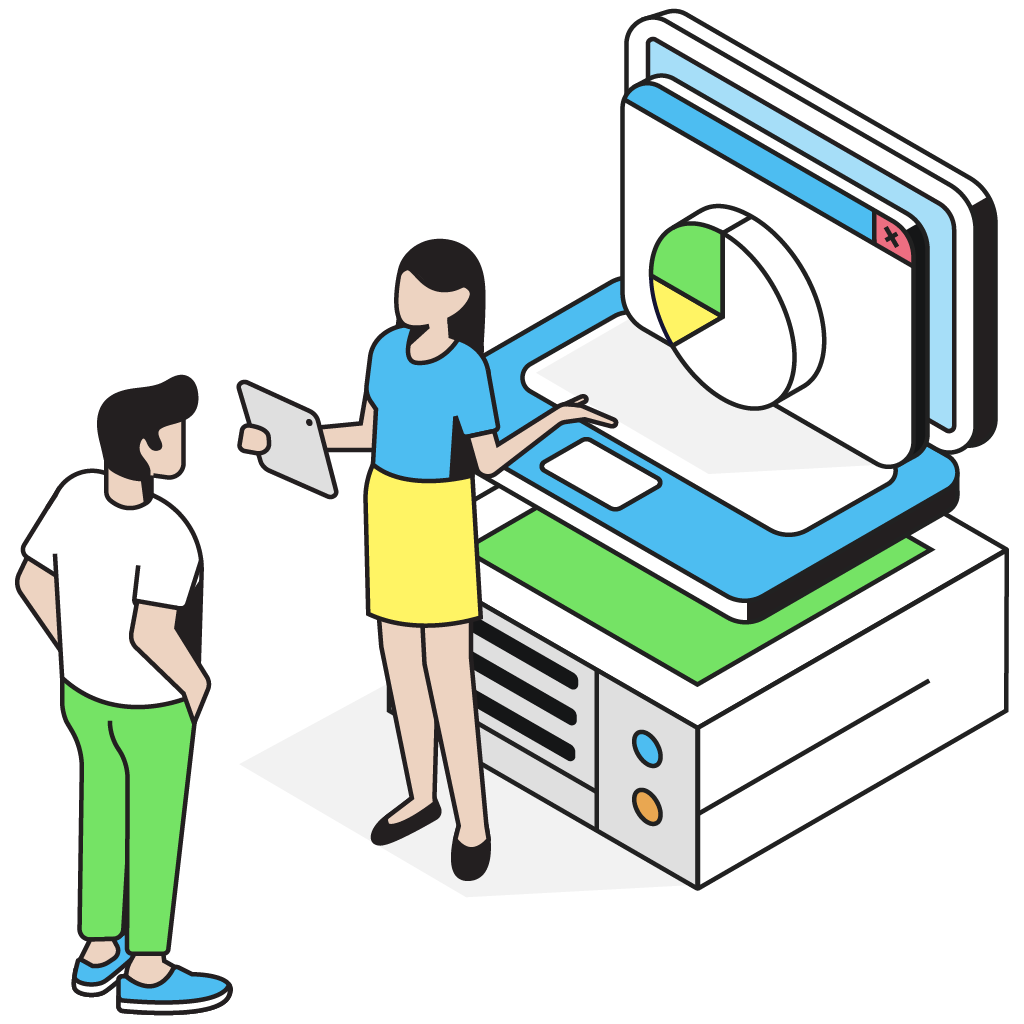
Manual intervention
Give domain experts more control
Enable non-technical data owners to have more input into data processes - so their knowledge of the data helps ensure downstream quality.
- Enable manual intervention into data pipelines to review, edit or approve data.
- Provide an added layer of data quality checks over and above automated validation.
- Maintain quality and control with built-in approval processes.
- Improve visibility and trust with full tracking of changes.

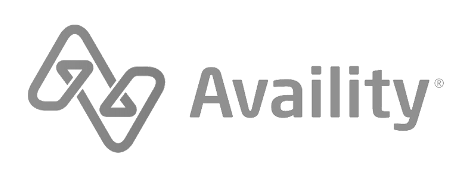
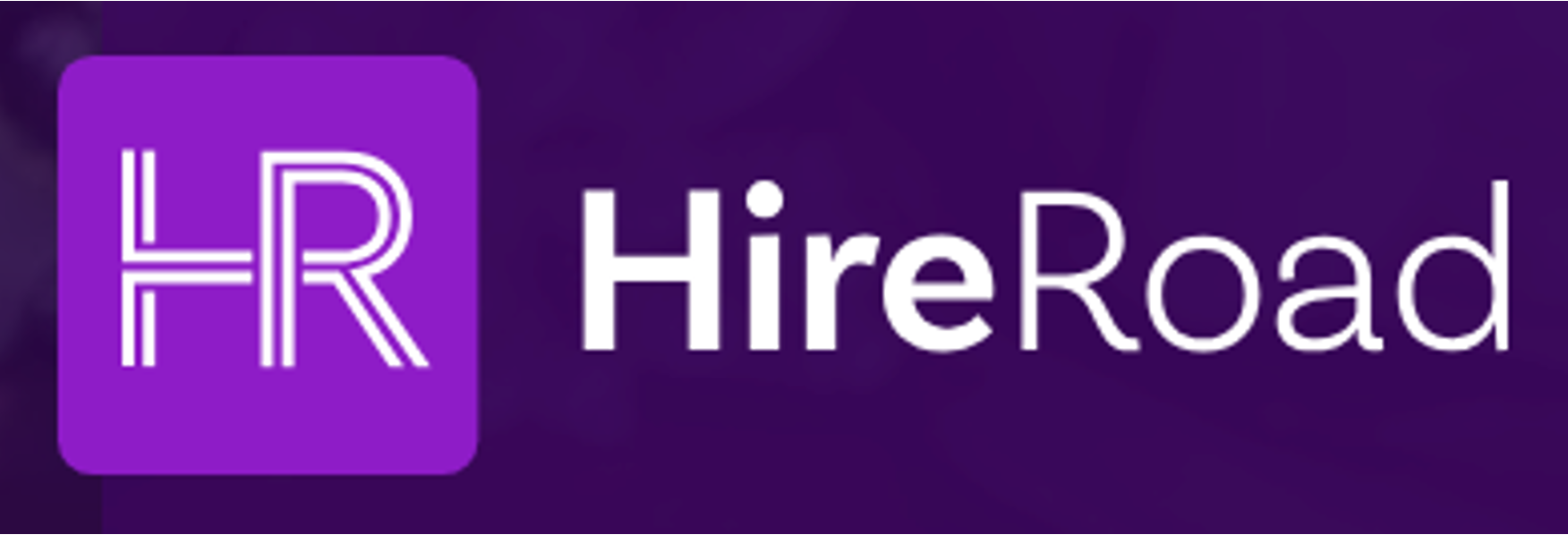
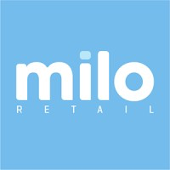
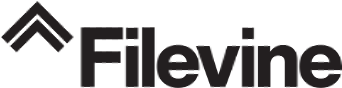
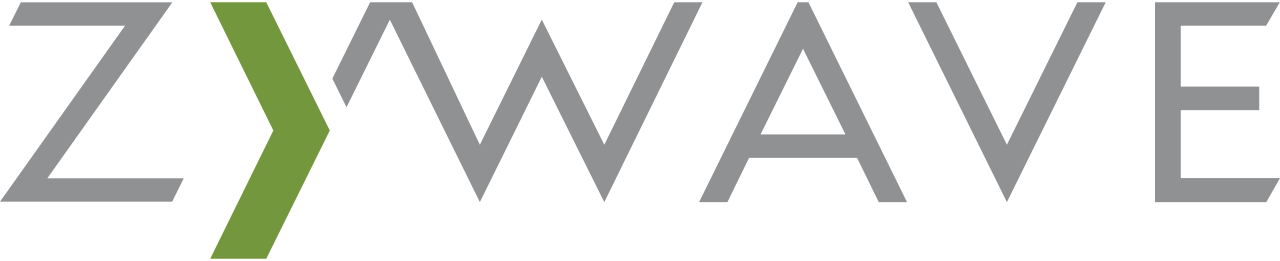
Master data management
Ensure high-quality reference data
Consistent, high-quality reference data is essential for trusted and unified outputs across your organization. With CloverDX, business users can own and control shared datasets, increasing accuracy and trust.
- Improve trust in data with a clear 'golden record'.
- Maintain consistency by automatically syncing changes between the master dataset and other business systems.
- Track all changes with full audit logs.
- Keep control with an approval process for any changes to master datasets.
Proactive error handling
Be on top of any issues
If you don't know there's a problem with your data workflows until someone else points it out, it leads to a lack of trust in the data and the process behind it.
When your data pipelines are built on CloverDX, you can have confidence that the data being delivered across the organization is accurate and reliable.
- Be first to know about problems with proactive alerts to any issues.
- Have peace of mind that everything is running ok, with always-on monitoring and a visual interface for data flows.
- Improve data quality with automated, always-on validation checks.
- Troubleshoot faster, with detailed error messages that show exactly what the problem is and where it occurred, and full audit logs of all your jobs.
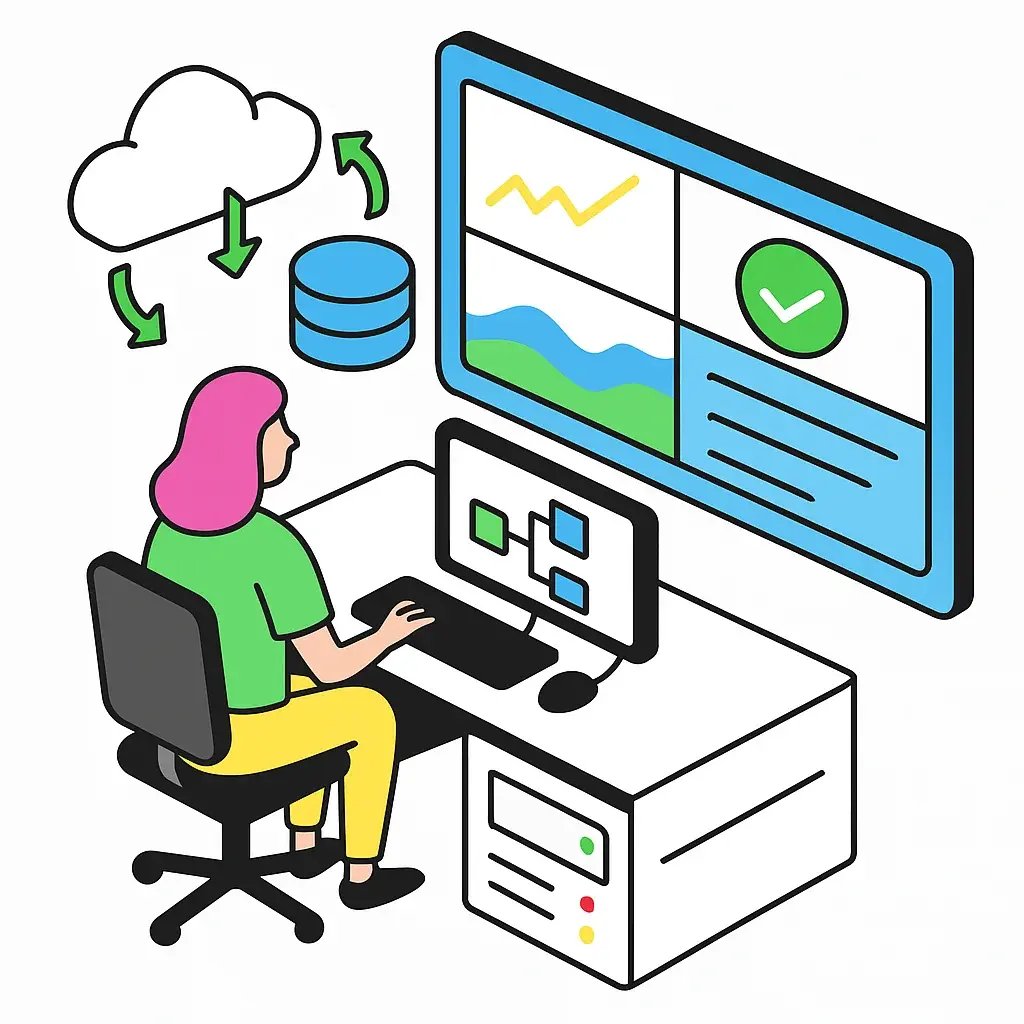
“CloverDX enables the team to easily see what's happening in the data as it flows through the process.”
- Nachalle Kortrink, Manager, BI and Information Provision, Van Mossel Automotive Group
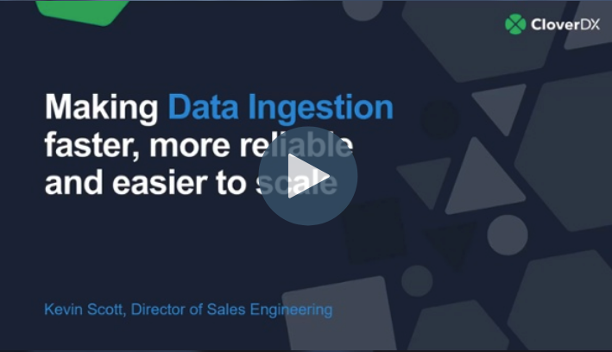
Webinar: Data ingest for faster onboarding
When your business is built on ingesting data from many customers, in many different formats, how do you scale up the volume of data and clients you can support – without adding headcount? See how you can automate data ingestion and reduce developer spend.
Data validation saves a logistics company over $800,000
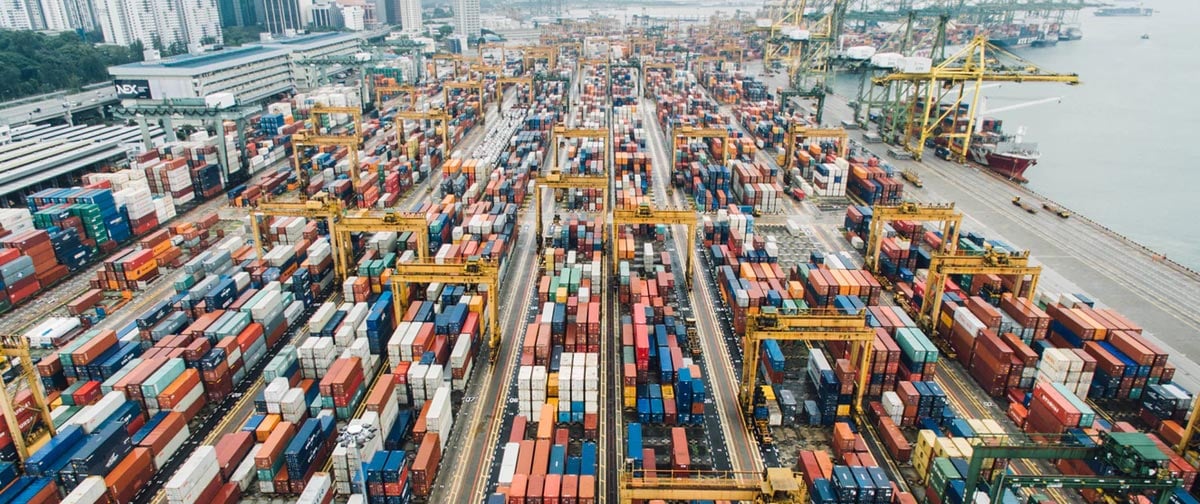
Automating data validation reduces manual intervention by 90%
The customer
A leading logistics company, which relies on accurate address information in multiple territories to optimize their processes.
The challenge
Regional differences in address structures and rules had required manual verification and correcting of data from a 30-person team, working shifts to meet strict delivery deadlines. This was a real bottleneck to the company's growth, and they needed to automated the data validation process in order to scale.
The results
A scalable address validation and cleansing framework, built in CloverDX, now validates and repairs 90% of addresses automatically, vastly reducing the need for manual human input.
- Elimination of manual input, with human intervention only needed in 10% of the cases it was before
- Hard-to-scale bottleneck is removed, allowing the company to grow without needing to hire more people
- Automated framework integrates with external data sources, for fast, accurate validation that can easily expand to new territories.
Implementing data validation in your data pipelines
Building pipelines to handle bad data reduces errors and increases user trust in the resulting data.
This post looks at some common sources of bad data, and how you can mitigate risk by implementing data quality measures at each stage of the pipeline.
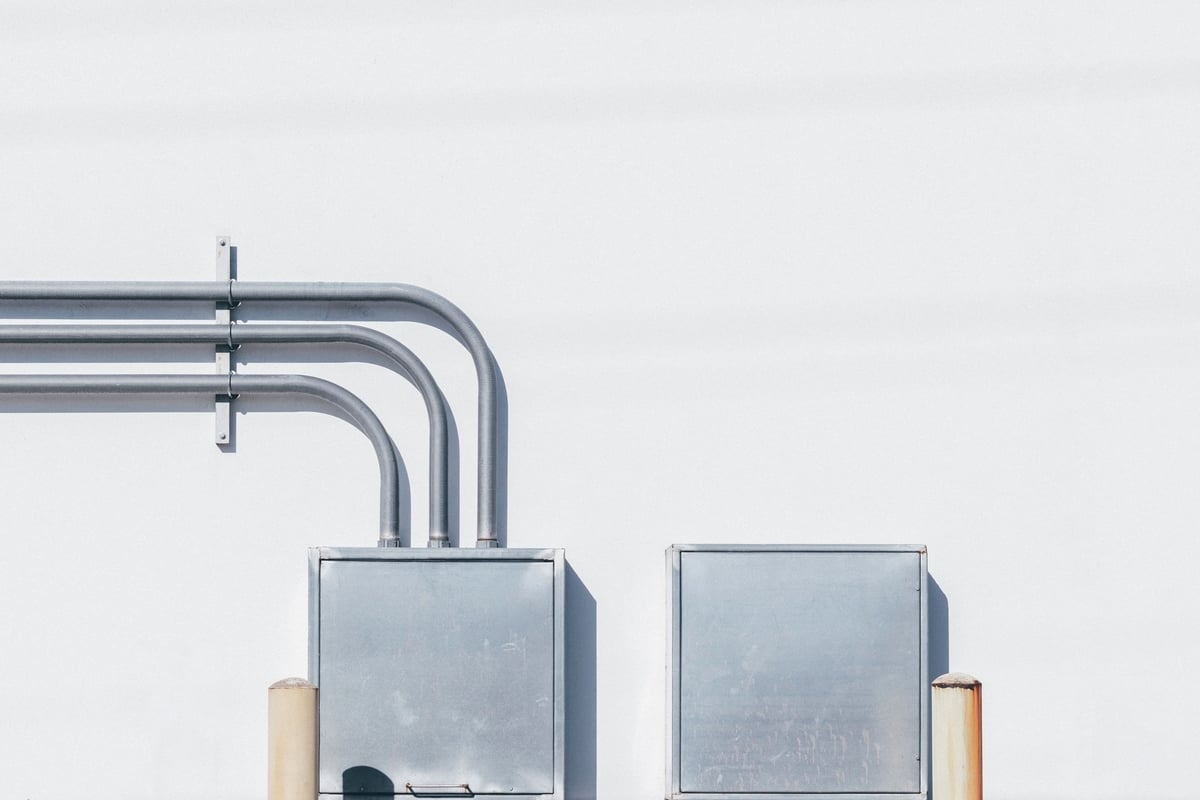
Building data pipelines to handle bad data
How to mitigate the risks of bad data in a data pipeline, including validation and profiling, and establishing an error management process.
Increase trust in your data with CloverDX
Request a demo and see how CloverDX can increase reliability, quality, and trust in data throughout your organization.